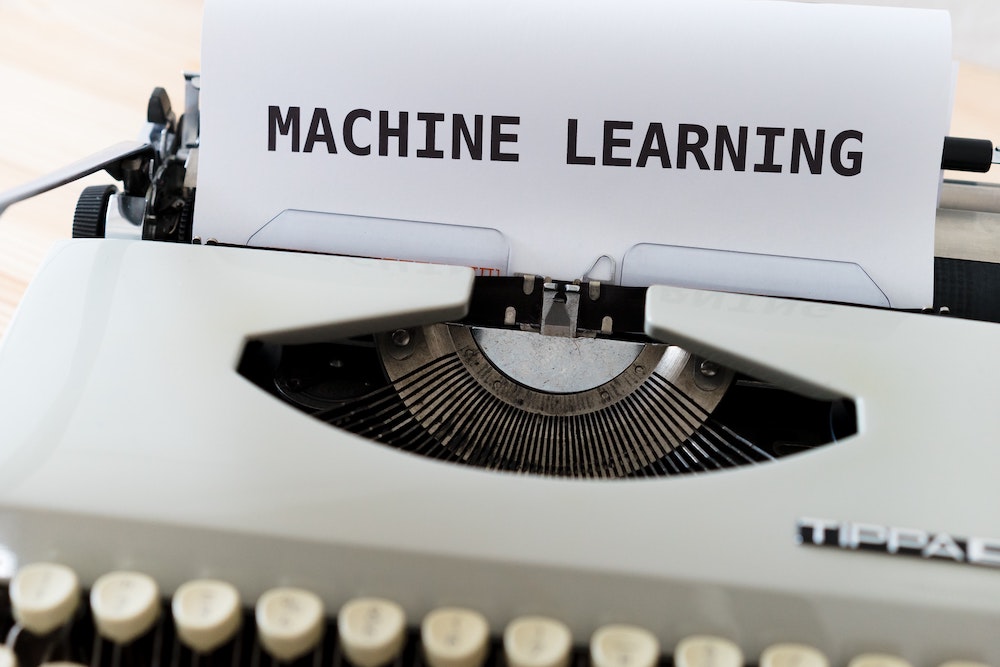
“There are perhaps two approaches to try to do quantum machine learning,” said Iordanis Kerenidis, senior vice president, quantum algorithms, QC Ware, in a recent briefing with HPCwire. “One is the heuristic approach, to use a quantum circuit and try to train it to do something; [it’s] the analog of deep learning or neural networks. You cannot prove many things, but you hope that quantum can offer something better than classical systems, right.
“The other option [is] looking at the mathematical procedures that you use in machine learning, like linear algebra and more complicated things like good domain optimization and subspace states like singular value decomposition. These [approaches] are at the core of machine learning. We try to understand what quantum can offer in these procedures and how we can apply them many different ways within machine learning.” QC Ware focuses on this latter approach.
In recent years, the quantum community has become a bubbling cauldron of new niches and companies. While most attention remains on the big (and small) quantum hardware developers chasing increased qubit counts and increased qubit/system fidelity – think IBM et al. – the quantum software developer community has grown in importance and visibility. QC Ware positions itself as a provider of hardware-agnostic “enterprise software and services for quantum computers.”
While these companies’ efforts vary in scope (workflow tools, application software, and consulting services), they are all busily building both pure-play quantum- and quantum-inspired software to take advantage of early NISQ quantum computers and existing classical hardware. QC Ware and similar start-ups such Zapata Computing, Aqnostiq and Terra Quantum have become front-line players in terms of early engagement with potential users. These companies are hardware-agnostic and the whole sector seems likely to become very competitive as early tire-kicking transitions from POC projects to production deployment.
QC Ware’s first product was QC Forge, a SaaS platform for exploring QC Ware algorithms and running them on various quantum hardware (Rigetti, IonQ, IBM Q Network, AWS Braket, and Nvidia). The company has since focused more on specific application areas – Kerenidis said, “We are evolving our products into two separate lines, one is for chemistry simulations, and one on the machine learning side.” – and its roster of customers/collaborators is growing and includes some impressive names such as BMW, Banco Itaú Unibanco, Airbus, Goldman Sachs, and Roche.
Steering clear of heuristic solutions, said Kerenidis, is one of QC Ware’s distinguishing features. Like most in the quantum community, QC Ware has embraced the idea of blended classical-quantum architectures as the likely end game. Now, during the NISQ era, it is developing both quantum-informed algorithms that run on classical hardware – think GPUs – and pure-play quantum algorithms that run on today’s NISQ systems. Underpinning all of these efforts, contends Kerenidis, is the company strength in mathematics.
“We started from what we already know and can mathematically prove, and try to get the ideas and intuition and turn them into solutions that you can explain why you think they will work better, and that you can also start using on NISQ machines. We did the same for the classical hardware. We started from what quantum can do, and what we can prove, and turn these mathematical ideas into something that you can do on a GPU as well – it’s not exactly the same thing because you need a quantum computer [for optimum performance], but we carry out the part that will still give you better performance on the classical computer,” said Kerenidis.
Currently, there’s plenty of discussion around chasing quantum advantage on the early crop of NISQ systems. Instead, QC Ware is emphasizing the use of quantum-inspired algorithms on classical systems. It is possible to achieve better-than-classical performance on existing hardware today, contends Kerenidis, who cited two ongoing QC Ware projects, one with Brazilian bank Banco Itaú Unibanco on a customer retention application and another with a big pharma on data generation in clinical trials. Both use quantum-inspired algorithms on classical hardware.
“Banco Itaú Unibanco came to us and said we want to predict which customers are planning to leave the bank so we can go and try to convince them to stay. We said, OK, we have this quantum idea which we know that the quantum computer could do very well in the future, but can we turn this idea into something that you can use today in the bank on existing hardware,” said Kerenidis.
“Actually, we provided a new machine learning model that improved their in-house model (the bank had an existing application) by 7%. It had been 71% and we went to 78% accuracy, which [translated] to hundreds of millions of reals (Brazilian currency) in income. Now on their site they are actually putting it in production. That’s something that started from these quantum ideas, but you can run today on classical hardware as well. So, this is the quantum product on the machine learning side.”
The second use case cited by Kerenidis involved coping with a common problem in clinical trials, which is missing data. These data gaps have many causes: the physician perhaps didn’t ask for the data; patients leave during the trial; instrument malfunction, etc.
“The main question they had is how do we fill in this missing data so that we can predict something much more accurately? This is a very common problem in machine learning, to generate data where it’s missing. They gave us what they’re doing today internally. The general technique is called data imputation,” said Kerenidis.
“Again, starting from quantum ideas and the idea that quantum can help diversify the data that one uses to train the models, we actually came up with a way that not only improves the predictions that they do, but also fills in the data in the same way every time. This [consistency] was very important to them for purposes of a clinical trial. Currently, this work is not in production use, but it’s an example of how we can improve vastly what they actually use in house, using quantum ideas. The prospect is to work more on that and make a real solution end to end solution for this problem.”
These quantum-informed solutions run on classical hardware are easier to implement as the computer architecture being used is familiar and often already in place. QC Ware, said Kerenidis, is leveraging its deep machine learning expertise, and growing domain knowledge. “You can have a generic algorithm, but if you want to solve a specific use case, you really need to go deep into understanding finance, like we’re working with finance or deep hedging. We need to understand everything about deep hedging before we can see what you can offer this particular thing,” he said.
QC Ware has produced several papers on its work. The most recent, Quantum Methods for Neural Networks and Application to Medical Image Classification, was published in Quantum in December and examines a joint project with Roche. (A list of a few recent works is at end of article[i].)
Broadly, QC Ware’s goal is to provide workflow tools and applications that will run on classical systems now, advancing quantum computers as they improve, and eventually on hybrid quantum-classical systems; the last method is how most observers now think quantum computing will become part HPC writ large. The Forge product allows users to experiment with QC Ware algorithms on existing quantum machines. QC Ware is now turning to domain solutions and custom solutions for clients. It also has active collaboration with several QC hardware developers.
IBM Eagle Quantum QPU
Kerenidis agrees that instances of narrow quantum advantage will likely pop up soon but thinks regular use of NISQ machines in production environments is still somewhat distant. Reminded that IBM plans to introduce a 1000-plus qubit QPU this year, he said, “I think what we need more than 1000 qubits is 200 good qubits. Right. So not error-corrected all the way, not perfect qubits, but I will be happy with 200 better qubits than 1000 qubits of the same quality that we have today. QC Ware is trying to not overhype quantum and be realistic about how things are going.”
Not surprisingly, QC Ware is working with most of the current qubit modalities to be ready as better quantum computers become available.
“We collaborate with PsiQuantum (photonic qubits) and had, for example a bilateral project between us, PsiQuantum and a big pharma company. We work more on understanding how to take our algorithms and make them optimal for their hardware. We haven’t used the hardware [yet] – we are trying to prepare ourselves. With Microsoft (topological qubits), the technology might be different, but they will be a similar type of machine as IBM or Google (superconducting qubits) in the sense that we don’t care about the hardware as long as they can do gates. If you give me a gate-based circuit, I can build my algorithm.”
The company has also worked with IonQ and trapped ion technology. It’s not yet worked much with neutral atom qubit technology (QuEra, Pasqal). “Because there is not so much algorithmic work that you can do on these machines yet. They don’t have gates. I see it as closest to the D-Wave machines,” said Kerenidis. QC Ware has worked with D-Wave, “on logistics or scheduling or these type of optimization problems. For example, we worked with a Japanese company, which is a Toyota affiliate (AISIN), on some logistics, problems we did use D-Wave.”
As a rule, the new batch of most quantum software companies don’t care about the underlying hardware beyond being able to run on it. Kerenidis thinks it’s not yet clear how competition between the differing qubit modalities will play out. Meanwhile, the company continues growing. Company headcount is around 50 now.
Kerenidis didn’t say much about the company’s Q2B conference initiative. The first one was in late 2017 and the last one was held in December 2022. It’s grown to a three times a year event held in the U.S, Japan, and France. The next one is in Paris in May.
“Q2B is a business-centric meeting, where companies showcase what they have been doing. It’s not that we don’t have scientific talks, we always have John Preskill (Caltech, coiner of the term NISQ) who will give the keynote and Scott Aaronson. So, we do try to connect with the technical side in the conference, but the primary objective is to see how quantum can actually make an impact in the business.”
Lot’s going on. Stay tuned
[i] Quantum Methods for Neural Networks and Application to Medical Image Classification
J Landman, N Mathur, YY Li, M Strahm, S Kazdaghli, A Prakash, …
Quantum 6, 881
Quantum Vision Transformers
EA Cherrat, I Kerenidis, N Mathur, J Landman, M Strahm, YY Li
arXiv preprint arXiv:2209.08167
Quantum machine learning with subspace states
I Kerenidis, A Prakash
arXiv preprint arXiv:2202.00054
Low depth algorithms for quantum amplitude estimation
T Giurgica-Tiron, I Kerenidis, F Labib, A Prakash, W Zeng
Quantum 6, 745
“The other option [is] looking at the mathematical procedures that you use in machine learning, like linear algebra and more complicated things like good domain optimization and subspace states like singular value decomposition. These [approaches] are at the core of machine learning. We try to understand what quantum can offer in these procedures and how we can apply them many different ways within machine learning.” QC Ware focuses on this latter approach.
In recent years, the quantum community has become a bubbling cauldron of new niches and companies. While most attention remains on the big (and small) quantum hardware developers chasing increased qubit counts and increased qubit/system fidelity – think IBM et al. – the quantum software developer community has grown in importance and visibility. QC Ware positions itself as a provider of hardware-agnostic “enterprise software and services for quantum computers.”
While these companies’ efforts vary in scope (workflow tools, application software, and consulting services), they are all busily building both pure-play quantum- and quantum-inspired software to take advantage of early NISQ quantum computers and existing classical hardware. QC Ware and similar start-ups such Zapata Computing, Aqnostiq and Terra Quantum have become front-line players in terms of early engagement with potential users. These companies are hardware-agnostic and the whole sector seems likely to become very competitive as early tire-kicking transitions from POC projects to production deployment.
QC Ware’s first product was QC Forge, a SaaS platform for exploring QC Ware algorithms and running them on various quantum hardware (Rigetti, IonQ, IBM Q Network, AWS Braket, and Nvidia). The company has since focused more on specific application areas – Kerenidis said, “We are evolving our products into two separate lines, one is for chemistry simulations, and one on the machine learning side.” – and its roster of customers/collaborators is growing and includes some impressive names such as BMW, Banco Itaú Unibanco, Airbus, Goldman Sachs, and Roche.
Steering clear of heuristic solutions, said Kerenidis, is one of QC Ware’s distinguishing features. Like most in the quantum community, QC Ware has embraced the idea of blended classical-quantum architectures as the likely end game. Now, during the NISQ era, it is developing both quantum-informed algorithms that run on classical hardware – think GPUs – and pure-play quantum algorithms that run on today’s NISQ systems. Underpinning all of these efforts, contends Kerenidis, is the company strength in mathematics.
“We started from what we already know and can mathematically prove, and try to get the ideas and intuition and turn them into solutions that you can explain why you think they will work better, and that you can also start using on NISQ machines. We did the same for the classical hardware. We started from what quantum can do, and what we can prove, and turn these mathematical ideas into something that you can do on a GPU as well – it’s not exactly the same thing because you need a quantum computer [for optimum performance], but we carry out the part that will still give you better performance on the classical computer,” said Kerenidis.
Currently, there’s plenty of discussion around chasing quantum advantage on the early crop of NISQ systems. Instead, QC Ware is emphasizing the use of quantum-inspired algorithms on classical systems. It is possible to achieve better-than-classical performance on existing hardware today, contends Kerenidis, who cited two ongoing QC Ware projects, one with Brazilian bank Banco Itaú Unibanco on a customer retention application and another with a big pharma on data generation in clinical trials. Both use quantum-inspired algorithms on classical hardware.
“Banco Itaú Unibanco came to us and said we want to predict which customers are planning to leave the bank so we can go and try to convince them to stay. We said, OK, we have this quantum idea which we know that the quantum computer could do very well in the future, but can we turn this idea into something that you can use today in the bank on existing hardware,” said Kerenidis.
“Actually, we provided a new machine learning model that improved their in-house model (the bank had an existing application) by 7%. It had been 71% and we went to 78% accuracy, which [translated] to hundreds of millions of reals (Brazilian currency) in income. Now on their site they are actually putting it in production. That’s something that started from these quantum ideas, but you can run today on classical hardware as well. So, this is the quantum product on the machine learning side.”
The second use case cited by Kerenidis involved coping with a common problem in clinical trials, which is missing data. These data gaps have many causes: the physician perhaps didn’t ask for the data; patients leave during the trial; instrument malfunction, etc.
“The main question they had is how do we fill in this missing data so that we can predict something much more accurately? This is a very common problem in machine learning, to generate data where it’s missing. They gave us what they’re doing today internally. The general technique is called data imputation,” said Kerenidis.
“Again, starting from quantum ideas and the idea that quantum can help diversify the data that one uses to train the models, we actually came up with a way that not only improves the predictions that they do, but also fills in the data in the same way every time. This [consistency] was very important to them for purposes of a clinical trial. Currently, this work is not in production use, but it’s an example of how we can improve vastly what they actually use in house, using quantum ideas. The prospect is to work more on that and make a real solution end to end solution for this problem.”
These quantum-informed solutions run on classical hardware are easier to implement as the computer architecture being used is familiar and often already in place. QC Ware, said Kerenidis, is leveraging its deep machine learning expertise, and growing domain knowledge. “You can have a generic algorithm, but if you want to solve a specific use case, you really need to go deep into understanding finance, like we’re working with finance or deep hedging. We need to understand everything about deep hedging before we can see what you can offer this particular thing,” he said.
QC Ware has produced several papers on its work. The most recent, Quantum Methods for Neural Networks and Application to Medical Image Classification, was published in Quantum in December and examines a joint project with Roche. (A list of a few recent works is at end of article[i].)
Broadly, QC Ware’s goal is to provide workflow tools and applications that will run on classical systems now, advancing quantum computers as they improve, and eventually on hybrid quantum-classical systems; the last method is how most observers now think quantum computing will become part HPC writ large. The Forge product allows users to experiment with QC Ware algorithms on existing quantum machines. QC Ware is now turning to domain solutions and custom solutions for clients. It also has active collaboration with several QC hardware developers.
IBM Eagle Quantum QPU
Kerenidis agrees that instances of narrow quantum advantage will likely pop up soon but thinks regular use of NISQ machines in production environments is still somewhat distant. Reminded that IBM plans to introduce a 1000-plus qubit QPU this year, he said, “I think what we need more than 1000 qubits is 200 good qubits. Right. So not error-corrected all the way, not perfect qubits, but I will be happy with 200 better qubits than 1000 qubits of the same quality that we have today. QC Ware is trying to not overhype quantum and be realistic about how things are going.”
Not surprisingly, QC Ware is working with most of the current qubit modalities to be ready as better quantum computers become available.
“We collaborate with PsiQuantum (photonic qubits) and had, for example a bilateral project between us, PsiQuantum and a big pharma company. We work more on understanding how to take our algorithms and make them optimal for their hardware. We haven’t used the hardware [yet] – we are trying to prepare ourselves. With Microsoft (topological qubits), the technology might be different, but they will be a similar type of machine as IBM or Google (superconducting qubits) in the sense that we don’t care about the hardware as long as they can do gates. If you give me a gate-based circuit, I can build my algorithm.”
The company has also worked with IonQ and trapped ion technology. It’s not yet worked much with neutral atom qubit technology (QuEra, Pasqal). “Because there is not so much algorithmic work that you can do on these machines yet. They don’t have gates. I see it as closest to the D-Wave machines,” said Kerenidis. QC Ware has worked with D-Wave, “on logistics or scheduling or these type of optimization problems. For example, we worked with a Japanese company, which is a Toyota affiliate (AISIN), on some logistics, problems we did use D-Wave.”
As a rule, the new batch of most quantum software companies don’t care about the underlying hardware beyond being able to run on it. Kerenidis thinks it’s not yet clear how competition between the differing qubit modalities will play out. Meanwhile, the company continues growing. Company headcount is around 50 now.
Kerenidis didn’t say much about the company’s Q2B conference initiative. The first one was in late 2017 and the last one was held in December 2022. It’s grown to a three times a year event held in the U.S, Japan, and France. The next one is in Paris in May.
“Q2B is a business-centric meeting, where companies showcase what they have been doing. It’s not that we don’t have scientific talks, we always have John Preskill (Caltech, coiner of the term NISQ) who will give the keynote and Scott Aaronson. So, we do try to connect with the technical side in the conference, but the primary objective is to see how quantum can actually make an impact in the business.”
Lot’s going on. Stay tuned
[i] Quantum Methods for Neural Networks and Application to Medical Image Classification
J Landman, N Mathur, YY Li, M Strahm, S Kazdaghli, A Prakash, …
Quantum 6, 881
Quantum Vision Transformers
EA Cherrat, I Kerenidis, N Mathur, J Landman, M Strahm, YY Li
arXiv preprint arXiv:2209.08167
Quantum machine learning with subspace states
I Kerenidis, A Prakash
arXiv preprint arXiv:2202.00054
Low depth algorithms for quantum amplitude estimation
T Giurgica-Tiron, I Kerenidis, F Labib, A Prakash, W Zeng
Quantum 6, 745